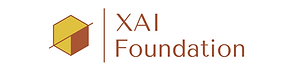
What is XAI?
Explainable AI (XAI) refers to the ability of an artificial intelligence (AI) system or model to provide clear and understandable explanations for its actions or decisions. In other words, XAI is about making AI transparent and interpretable to humans.
​
In many cases, AI models are trained using complex algorithms and large datasets, which can make it difficult for humans to understand how the model arrived at a particular decision.
This lack of transparency can create problems in areas such as healthcare, finance, and law, where decisions made by AI systems can have significant real-world consequences.
XAI seeks to address this issue by developing AI models that can provide clear and understandable explanations for their decisions. This can help increase trust in AI systems and ensure that they are used appropriately and ethically.
Some techniques used in XAI include generating textual or visual explanations for model outputs, identifying the most important features that influenced the model's decision, and providing interactive interfaces that allow users to explore the model's behavior.
​
What is the relationship between explainability and accuracy?
The relationship between AI explainability and accuracy can be complex and context-dependent.
​
In some cases, explainability and accuracy can be complementary. For example, when using AI for decision-making in fields such as medicine or finance, it may be important to have explainable models that provide a clear rationale for their recommendations or decisions. In such cases, an explainable model can also help improve accuracy by allowing human experts to identify and correct errors or biases.
​
​
On the other hand, there may be situations where explainability and accuracy are at odds with each other. For instance, some complex AI models, such as deep neural networks, can achieve high accuracy but may be difficult to explain in a way that humans can understand. In such cases, trade-offs may need to be made between accuracy and explainability, depending on the specific use case and the level of importance placed on each.
In general, it is important to strike a balance between accuracy and explainability when developing AI systems, taking into account the needs and requirements of the intended users and the specific context in which the system will be deployed.
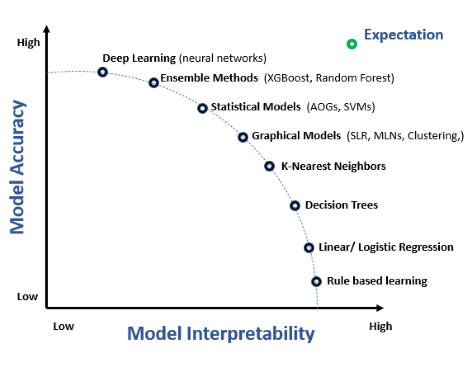
Source: Arya AI Blog
Why is XAI Important?
XAI is important for several reasons:
-
Transparency: XAI makes it easier to understand how AI algorithms make decisions. This can help build trust in AI systems, especially when they are used to make important decisions that affect people's lives.
-
Accountability: XAI can make it easier to identify errors or biases in AI algorithms, which is important for holding developers and users accountable.
-
Compliance: XAI can help ensure that AI systems comply with regulations such as the General Data Protection Regulation (GDPR) and other privacy laws.
-
Safety: XAI can help identify potential safety risks associated with AI systems, such as self-driving cars or medical diagnosis tools, which can help prevent accidents or harm to users.
-
Innovation: XAI can foster innovation by making it easier to understand and improve AI systems, which can lead to better performance and more advanced AI applications.
Overall, XAI is important because it can help ensure that AI systems are transparent, accountable, compliant, safe, and innovative.
What is the future of XAI?
The future of XAI (explainable artificial intelligence) is likely to be shaped by several key trends and developments:
​
-
Increased demand: As AI systems become more prevalent in various industries and applications, there is likely to be an increasing demand for XAI to improve transparency, accountability, and trustworthiness.
-
Advancements in technology: Advances in machine learning and related technologies, such as natural language processing and computer vision, are likely to lead to new methods and tools for XAI that enable more accurate and interpretable models.
-
Regulatory requirements: Governments and industry regulators are beginning to require more transparency and explainability in AI systems, which is likely to drive further development and adoption of XAI.
-
Interdisciplinary collaboration: Collaboration between researchers in different fields, such as computer science, psychology, and human factors engineering, is likely to lead to new insights and approaches for XAI.
-
Ethical considerations: As AI systems become more powerful and influential, there is likely to be an increasing focus on the ethical implications of their use, which is likely to drive further research and development of XAI.
Overall, the future of XAI is likely to be characterized by continued growth, innovation, and collaboration across different disciplines and industries, driven by the need for more transparent, accountable, and ethical AI systems.